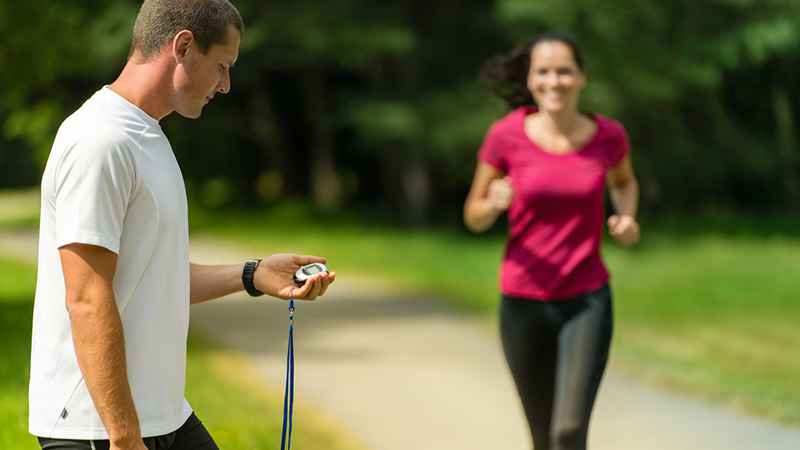
“There is increasing concern that most current published research findings are false.”
— Dr. John Ioannidis, Stanford University School of Medicine
It’s the nature of science to be always changing. But it’s not nature that changes so much as the ability of science to make sense of it.
Thanks to observations and research, there are ongoing modifications, adjustments, overhauls and tweaks that are seemingly endless in the scientific world. But a weak link in the science has led to questionable research results of major proportions. Let’s look at the problem, the players and some possible solutions to this serious issue.
The Problem
The Ioannidis quote above points out the obvious problem. When many well-respected scientists have been telling the world that most published research may be flawed, everyone should listen and act.
In the 1950s, scientists adopted a method called null-hypothesis significance testing (NHST). What seemed like an objective way to draw conclusions from research data became popular, and today almost all empirical research is guided by it, along with p values — now referred to as tricky conditional probabilities that few understand correctly. The lure of this “old statistics” approach is the notion of “statistical significance,” but it became apparent that this method can be dead wrong.
Among the many research examples was the drug Vioxx, demonstrated safe through old statistics. It became a leading pain-relief medication until increased reports of heart attacks, strokes and death. Finally, it was removed from the market in 2004 after five years and billions of dollars in sales, and the uncovering of the real statistics demonstrating its danger.
The old standard of statistical significance may in fact be tiny and trivial, and sometimes interpreted as “pretty close,” notes Geoff Cumming of the Statistical Cognition Laboratory, School of Psychological Science, La Trobe University in Australia.
The old statistics aim to “freeze, quantify and package uncertainty — that fundamental imponderable of human existence,” Aleksandar Aksentijevic recently wrote in “Statistician, heal thyself: fighting statophobia at the source” (Frontiers in Psychology 2015).
Sometimes, as Tatsioni et al. (JAMA 2007) state, the same data are used and interpreted entirely differently by different investigators depending on whether they supported findings from the studies.
It should be no surprise that outdated exercise science can lead to a lack of consensus as well. After decades of research and billions of dollars spent, there is still disagreement on many of the most basic issues regarding training, eating and performance. This involves the application of the foundations of exercise science such as VO2max, lactate, running economy and max heart rate. Midgley et al. (2007) state that, “consequently, it would be difficult to argue against the view that there is insufficient direct scientific evidence to formulate training recommendations based on this limited research.”
So what happens to all the studies with questionable or flawed results? Once published — the scientific stamp of approval — other researchers sometimes use and reference them. Even if the original study was proven wrong, it remains in the scientific literature.
Scientists are obviously not the only ones affected by this problem. Other players in the exercise field are too. Clinicians and coaches read the studies, and athletes themselves do too, through the media (which often misrepresents or distorts studies — another problem altogether), not to mention students. Flawed research results can literally impede the progress of health and fitness, or worse.
The Players
The core players in exercise science are those most affected by the problem defined above:
- Clinicians working regularly with athletes continually evaluate each individual’s physical, biochemical and mental-emotional condition in a holistic way. Training and competitive schedules, any and all test results, dietary habits, footwear and other factors all influence short- and long-term performance. This helps clinicians recognize and respond to abnormal signs and symptoms with appropriate modifications that remove impediments that can impair progress while maintaining health. Some clinicians are capable of implementing such approaches, serving as doctor, nutritionist, physical therapist and coach, for example. However, in many cases more than one liked-minded professional “team” is effective. This excludes clinicians in specialties such as surgery, endocrinology and others but can include scientists.
- Scientists are academic-oriented professionals interested in exploring human performance with precision, properly gathering data to interpret or theorize outcomes in published studies. By presenting the experiences of athletes, including extensive test and performance results, scientists can add to our knowledge of both health and fitness, and can work together with clinicians and athletes to better understand exercise science.
- Athletes are those individuals who are elite and age-group competitors, those who go to the gym twice a week or walk each morning, those just embarking on a healthier lifestyle and even coach potatoes not yet ready to realize their human potential. In essence, everyone is an athlete.
Another player in the game are the companies who fund many of the studies. Manipulating old statistics is not difficult, while much of the research that goes against their financial interests is never published and remains secret.
Clinicians and scientists sometimes appear to be at odds with each other’s work and for good reason. Clinicians (those who avoid cook-book formulas) individualize their approach and are more empirical in their evidence-based methods. They may use scientific reasoning to explain what they do; however, research does not drive the process in most cases. Clinical opinions don’t come with numbers, yet scientists want them for scientific explanations.
While this may appear like an impasse, it’s really an opportunity to work more closely, which would benefit athletes too.
The Solutions
The “new” statistics can help exercise science improve research quality, possibly leading to a consensus of important concepts, and the real potential for more collaborative work among all players.
Cumming states, “We need to make substantial changes to how we conduct research. First, in response to heightened concern that our published research literature is incomplete and untrustworthy, we need new requirements to ensure research integrity.” These changes, he says, will prove valuable: “Our publicly available literature will become more trustworthy, our discipline more quantitative, and our research progress more rapid.”
In the last 10 years, leading scholars have mounted what could be called a revolution to address the problem, proclaiming the deep flaws of NHST and how it damages research progress. These experts instead advocate what is called estimation, meaning effect sizes and confidence intervals as statistical tools that are honest, accurate and informative ways to analyze and present data — the “new statistics.”
In 2015, the journal Basic and Applied Social Psychology banned p values and other traditional statistical analyses. Instead, they encourage the use of larger sample sizes, because descriptive statistics become increasingly stable and sampling error is less problematic. The editors state that sometimes p values serve as an excuse for lower quality research, emphasizing that the popular null hypothesis significance testing procedure is invalid. They anticipate this will eliminate an important obstacle to creative thinking. Other journals will follow suit.
Current training methods have developed predominantly from the trial-and-error approach, sometimes through the more careful experimentation, of clinicians, coaches and athletes, while the contributions made by scientists have been comparatively small. This lack of available scientific knowledge becomes a great potential for collaborative activities among scientists, clinicians and athletes.
Clinicians and the ‘new’ statistics
More than the traditional scientific methods, clinicians described here think and work like the new statistics. An example is meta-analytic thinking. Meta-analysis is the statistical procedure scientists use to combine data from various studies. Clinicians, however, don’t use any one study, or more importantly, the experience of a single athlete’s response to their care, but rather build an approach based on all past clinical experiences. Other examples of new statistic guidelines modified for clinical application, include:
- Promote integrity.
- Understand and share outcomes with other clinicians.
- Avoid dichotomous thinking, especially when teaching athletes.
- Always search for better techniques.
- Keep things simple, in most cases. While the human body is extremely complex, teaching athletes about health and fitness should be easy enough to implement recommendations successfully.